From Good AI to Good Data Engineering. Or how Responsible AI interplays with High Data Quality
Oct 30, 2024
•
Thomas Kranzkowski
Responsible AI depends on high-quality data engineering to ensure ethical, fair, and transparent AI systems.
The intersection of artificial intelligence (AI) and data engineering has become increasingly critical. As AI technologies proliferate, the need for responsible AI — AI that is ethical, transparent and fair — has never been more pressing. Central to this concept is the quality of the data these AI systems are built upon. This article explores the necessity of responsible AI, its key aspects and how it inherently relies on high data quality within data engineering, offering a glimpse into the future.
Responsible AI on the Rise
We can speak no longer about AI as having the potential to revolutionize industries, improve efficiencies and enhance our daily lives, it already does! However, with great power comes great responsibility (Voltaire, having philosophy in school can be helpful here). Responsible AI is about ensuring that AI systems are developed and deployed in ways that are ethical, transparent and fair. Without responsible AI, there is a risk of perpetuating (keeping) biases, violating privacy and making mistaken decisions that could have significant consequences for individuals, society and whole businesses.
The importance of responsible AI cannot be overstated enough. As AI systems become more integrated into critical decision-making processes — such as hiring, medical diagnosis and criminal justice — the stakes are high. Guaranteeing that these systems operate responsibly is crucial for maintaining public trust and leveraging AI’s full potential.
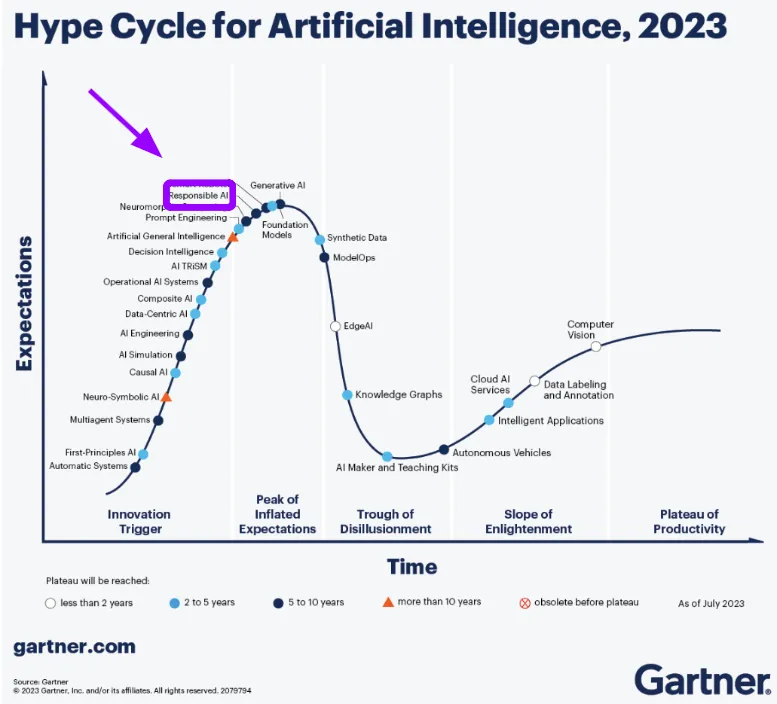
What means Responsibility in AI?
The word “Responsible” in Responsible AI refers to the ethical and conscientious approach to the development, deployment, and usage of AI technologies. It comes from the need to ensure that AI systems are designed and operated in ways that are fair, transparent and beneficial to society. The roots of this concept:
Ethical Considerations: AI should align with ethical standards, ensuring that its applications do not harm individuals or society. This involves adhering to principles such as fairness, transparency and accountability.
Transparency: AI systems should be understandable and explainable. Stakeholders, including users and regulators need to comprehend how decisions are made to trust and validate these systems.
Fairness: AI should be designed to prevent bias and ensure equitable treatment. This involves using diverse and representative data sets to train AI models and continuously monitoring for discriminatory outcomes.
Accountability: There should be mechanisms in place to hold AI systems and their creators accountable for their actions and decisions. This includes having clear lines of responsibility and ways to seek remedy.
Privacy and Security: An AI system must safeguard the privacy and security of data. This involves implementing robust data protection measures to prevent unauthorised access and misuse.
Key Aspects of Responsible AI
Implementing responsible AI involves several critical aspects. These are essential to ensure that AI systems. By focusing on these aspects, organizations can build AI systems that are not only technologically advanced but also ethically sound and trustworthy:
Bias Mitigation: Identifying and mitigating biases in AI models is essential for fair outcomes. This requires continuous monitoring and updating of models to address potential biases that may arise.
Explainability: Developing AI systems that can provide clear and understandable explanations for their decisions is crucial for transparency. This helps build trust and allows stakeholders to assess the system’s fairness and accuracy.
Robustness and Reliability: AI systems should be robust and reliable, performing consistently well across different scenarios and conditions. This ensures that they can be trusted to make accurate and dependable decisions.
Ethical AI Development: AI developers should follow ethical guidelines and best practices throughout the development process. This includes considering the societal impact of AI applications and ensuring they align with ethical standards.
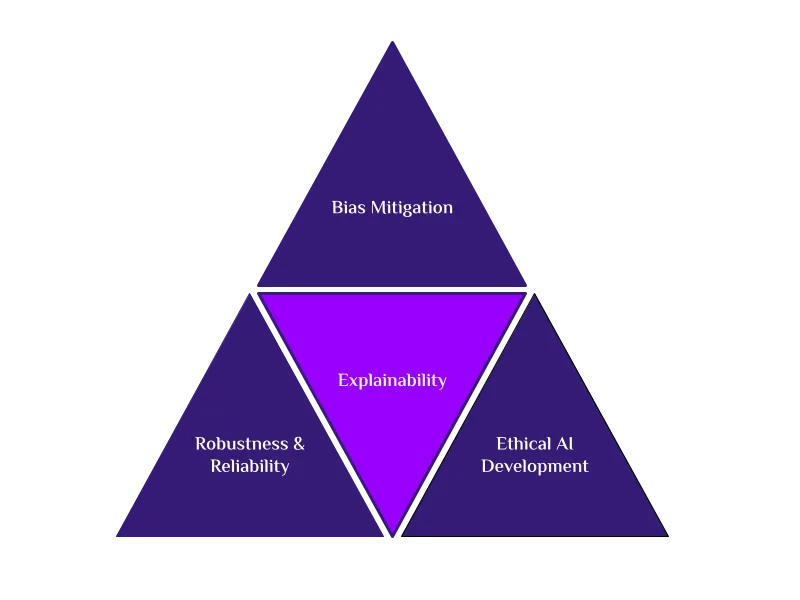
The trustworthy Quartett
Bridge over troubled Quality
High data quality is the bedrock of responsible AI. The quality of data used to train AI models directly impacts their fairness, accuracy and reliability. Data engineering plays a pivotal role in ensuring high data quality by implementing processes and practices to manage, clean and validate data.
Just to name a few key practices in data engineering that support high data quality include:
Data Cleaning: Removing errors, inconsistencies and duplicates from data sets to ensure accuracy and reliability.
Data Validation: Implementing validation checks to ensure data meets predefined standards and is fit for use.
Data Integration: Combining data from multiple sources to provide a comprehensive and consistent view.
Data Governance: Establishing policies and procedures to manage data quality, security, and compliance.
By focusing on these practices, Data Engineers make sure that the data used in AI systems is of high quality, supporting responsible AI development.
So, what next?
The future of AI and data engineering is intertwined, with responsible AI and high data quality at the spearhead. As AI technologies evolve, the demand for ethical, transparent and fair AI will continue to grow. Data engineering will play a critical role in meeting this demand by ensuring that data is managed, cleaned and validated to the highest standards.
In conclusion, the interplay between responsible AI and high data quality is essential for developing AI systems. As we move forward, focusing on responsible AI, robust data engineering practices will be key to harnessing the full potential of AI while safeguarding the interests of individuals and society.
Latest
Portable by design: Rethinking data platforms in the age of digital sovereignty
Build a portable, EU-compliant data platform and avoid vendor lock-in—discover our cloud-neutral stack in this deep-dive blog.
Cloud Independence: Testing a European Cloud Provider Against the Giants
Can a European cloud provider like Ionos replace AWS or Azure? We test it—and find surprising advantages in cost, control, and independence.
Stop loading bad quality data
Ingesting all data without quality checks leads to recurring issues. Prioritize data quality upfront to prevent downstream problems.